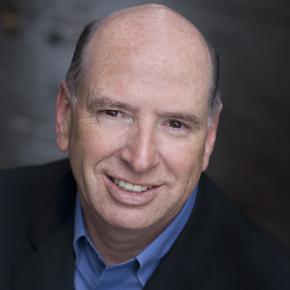
Patrick Culhane
- Principal architect of FICO® credit-scoring franchise during 15-year career with Fair Isaac Corporation, inventors of the FICO credit scoring system.
- EVP-Financial Services with global responsibility for Fair Isaac's dominant business line worldwide.
- 12 years in private consultancy in marketing and credit analytics, and provision of expert witness services in credit score-related litigation.

- All 7 Best Practices
- Pre-Meeting Discovery Process
- One-on-One Call with Expert
- Meeting Summary Report
- Post-Meeting Engagement
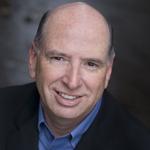
Predictive Analytics Strategy
Common Problems
- Accessing, assembling and putting development data into a usable state is a perennial challenge.
- There may be vast amounts of potential modeling data in your organization, but that doesn’t mean you can easily get your analytic hands on it. Barriers and limitations can abound, even in data-rich organizations. And often there are data gaps that need filling – areas where licensing or purchasing data is a requirement. It is therefore common that the costliest component (in time and money) of an analytic project is associated with assembling the right data for the task.
Modeling always makes use of historical “predictive” data – the data on which predictions are based – and “outcome” data – the data representing the result or event you are attempting to predict. Both of these must be present in sufficient quantities for analytic efforts to move forward.
Data sets are often missing observations. In some circumstances, “missing” might carry a meaning all its own, in other circumstances it may not. The analyst must determine if a missing observation has importance and if so, how it will be treated in predictive work.
Data obtained from multiple sources must often be linked, but if the “key” that links the sources is ambiguous, the linkage may fail. Sometimes the sources do not line up “one to one.” The collection time frames for the various data sources may not be consistent. These types of circumstances call for understanding and judgment calls about what is the appropriate course for the modeling effort.
Analysts can become hamstrung, and efforts slowed significantly, when the right development data is not available. And “right” not only means having potential for predictive use, but also how it is transformed for use in your analytic environment.
- There are many new tools for analysis, but the tools can get in the way of fully understanding the data.
- Recently I watched a video demonstration of a popular visualization tool – a tool that takes data and makes a picture out of it. During the demo, some of the data points identified as “outliers,” muddying up an otherwise pretty picture. The video showed how easily these outliers could be erased by simply circling them and swiping them off the screen. The action cleaned up the picture a lot.
So it might be really tempting to simply erase the outliers. But any good analyst would want to dig into those outliers to find out why they're there. Questions they may ask are:
- Are they symptomatic of relationships not discovered yet?
- Do they represent measurement changes or errors that may bleed through to other data, perhaps not so visibly?
One of the things an analyst gains from the modeling exercise is an understanding of how the data fundamentally relates to whatever they are trying to predict. The beauty of these tools is that they can do a lot in a very short period of time. The liability of them is that they can do a lot in a very short period of time and because of that, some of those important kinds of insights might never happen.
Organizations certainly need the tools, but they need to develop the right sort of professional culture so they can take advantage of the tools and still gain those important insights. - Some companies aren't sure where to start with predictive analysis.
Especially for the smaller organization, a common problem is knowing where to start. If you believe you can leverage predictive analytics and you're not, or you're doing it at a very crude level, you face a few questions:
- Where do I go from here?
- Where do I get started?
- What sort of elements does my company have that are proprietary?
- What data do we have that is unique to us?
- What data might provide significant value if only they were leveraged in an analytic framework?
- How do we create some sort of commercial value out of predictive analytics in that environment?
In some industries and settings where the technology is still just being adopted, initial efforts in predictive analytics can yield some big rewards. Educational institutions and fundraising organizations are areas where there is a relatively new use of predictive analytics. But in other industries, where the competitive environment is more advanced, a much larger investment is needed to enter, because analytics represents a formidable competitive moat.
A company trying PA for the first time might be hesitant to take the first step because it thinks it may not be big enough. But, in reality, it is taking the step that's important, not how big a step it is to start.- It can be very difficult to recruit analytical talent.
Analytic talent tends to be scarce. Advanced education focused in the field is relatively new, and the base of experienced professionals is low relative to the demand.
Until the last decade there were no advanced degree programs in predictive analytics. Earlier practitioners were often from the areas of statistics, operations research and related areas of applied mathematics. Fresh graduates from these disciplines could be recruited and then schooled and molded in the particulars of predictive analytic efforts “on the job.” Over the course of years they would also develop expertise in the domain of their practice – for example, banking, financial services or health care.All that is changing. Today, there are 49 masters degree programs in predictive analytics in the U.S., The oldest of these programs began in 2007, and 19 of them graduated their first class in 2014. This growth is evidence of the heightened demand for the specialized skills necessary to drive PA success. These degree programs allow companies to identify graduates who already have education in the particulars of predictive analytics.
Experienced practitioners in highly developed industries (such as banking and health care) are also a rare commodity. For managing the complex interactions of analytic efforts, one often needs experienced talent. So finding the right individuals with the combination of analytic background and business acumen, in the face of growing demand for these skills, is a big challenge.- Implementing predictive models can be as much or more work as developing them.
You’ve acquired and assembled the data and talent necessary to build models, and the projected results are stellar. At this point, though, your work may be far from done.
In most cases, the models need to be programmed and tested in the environment where they will have an impact on business decisions. Treatments need be assigned to the various model ranges. Perhaps some new treatments will affect customer service approaches and projected inbound call volumes. If you are in a regulated industry, all of these changes may be subject to regulatory scrutiny. Finally, the performance of the models needs to be tracked to ensure the models are accurately predicting the outcomes intended in the real world, and adjustments made if and when performance slips.
Companies can underestimate the effort required to implement predictive models in the setting that delivers decisions and business results. And many analytic projects fail, or result in wasted efforts, because they do not anticipate the implementation hurdles.